A keynote by Pia Andrews
Conversations from CLAWCON 2023
From 12 to 14 July 2023, the Singapore Management University’s Centre for Computational Law (“SMU CCLAW”) hosted the 1st Edition of the Computational Law Conference (“CLAWCON”), at the SMU Connexion Event Square. The inaugural conference was structured around the theme of “Computational Law + Symbolic AI + Industry Adoption challenges, issues, and real-world implementations”. This article distils the key points from Pia Andrews’s keynote on the topic of “Government-Citizen Interactions & Computational Law”.
This is the second part of the article. It is recommended that you first read the previous part:
Potential enablers for a modern “shared policy infrastructure”
Andrews proposes a few potential enablers to create a modern “shared policy infrastructure”. Examples include the introduction of:
- public engagement and modelling tools (which aid to explore and test options and modelling of impact) to support test-driven policy ideation;
- a public repository (consisting of policy tools, government models, measurement frameworks, synthetic population data, etc) to support test-driven policy development & drafting;
- a policy twin (the policy equivalent of a “digital twin”, or reference implementations of “policy as code” such as api.legislation.gov.xx) to support lawful policy interpretation, implementation and delivery; and
- escalation and policy iteration mechanisms (which aid to reveal and act upon dark patterns at portfolio and whole-of-government levels) to support policy compliance, iteration and improvement over time.
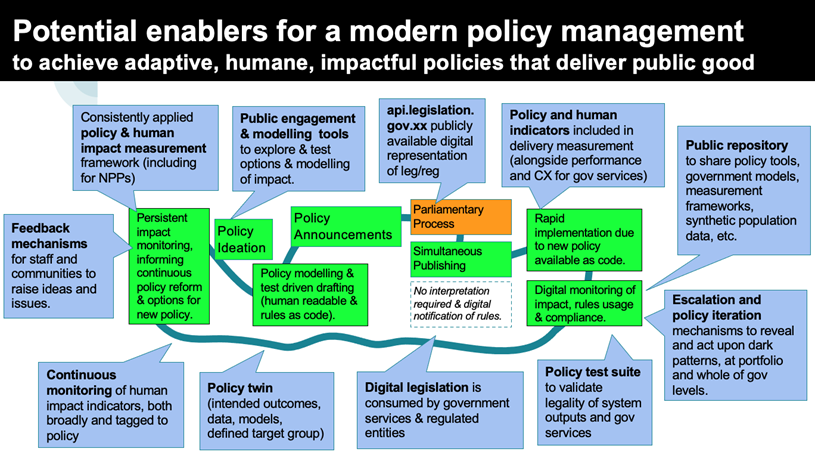
Overall, Andrews believes that a “shared policy infrastructure” can drive purposeful, test-driven, lawful, adaptive and humane public services & policies in the following ways.
(1) A shared policy infrastructure can drive purposeful public services
First, it is vital to remember the original purpose of the public service in question, and to avoid placing sub-goals above this purpose. Unfortunately, this is challenging because goals tend to be more easily quantifiable than purpose, and humans henceforth tend to gravitate towards relying on the former, instead of the latter, as yardstick(s) to measure the success of public services. Given this trend, all outputs of public services should be aligned to their intended outcomes to provide purposeful, rather than perverse, incentives.
Andrews proposes the following tips to make public services more purposeful:
- Ensure all policies, legislation, regulation, programs, projects and services codify, measure and monitor for the intended policy outcomes.
- Outcomes based funding and structures.
- Regular cadence to review effectiveness of all policy interventions (including services).
(2) A shared policy infrastructure can move guess-driven policy development to a test driven one
Second, policy development should be test-driven rather than guess-driven because a trial-and-error testing approach is essential in crafting solutions, and pure reliance on evidence is not ideal in this aspect.
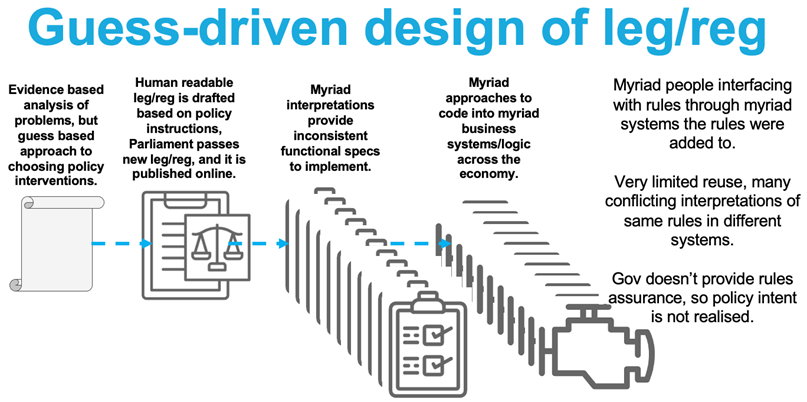
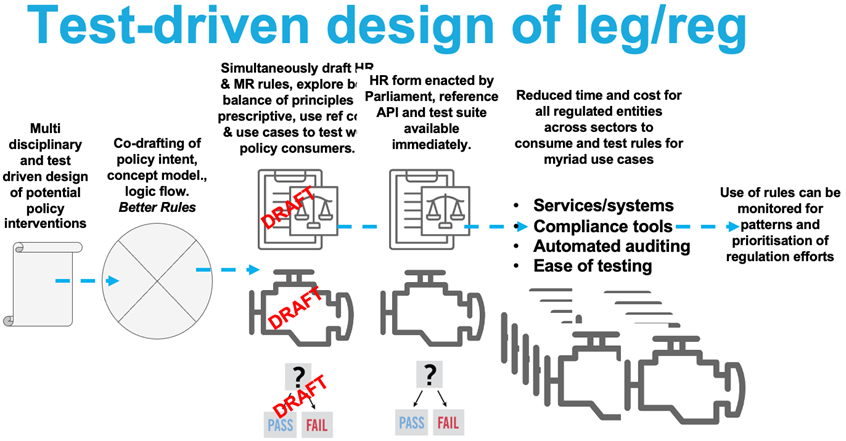
Andrews proposes the following tips to make policy development test-driven:
- Test-driven development of policy purpose.
- Test-driven exploration of effective policy interventions.
- Test-driven drafting (human & machine readable).
- Baseline and monitor purpose measures & indicators.
- Ensure funding bids identify & drive purpose/policy.
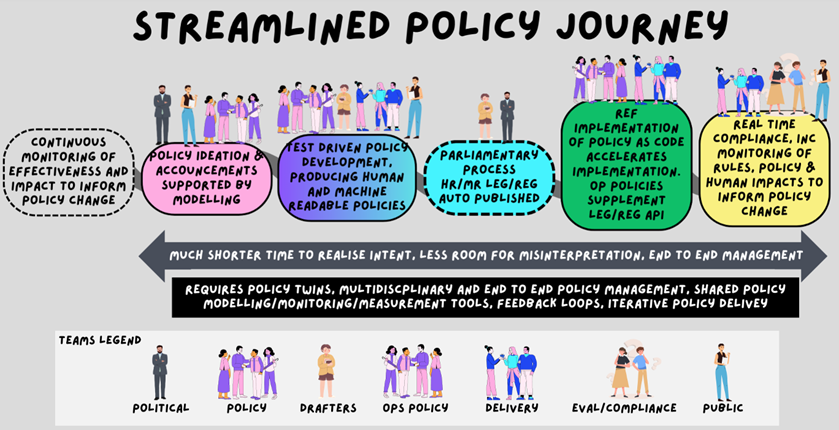
(3) A shared policy infrastructure might make government services more lawful
Third, it is important to distinguish legislation from operational policies and system constraints because the latter two may unintentionally render government services unlawful in the absence of CLAW or legislation as code (“LaC”). For example, although a legislation may stipulate that the benefits to be paid out are A + B = C, operational policies and system constraints may result in a miscalculation of the benefits to be paid out as (A + B) / 2 = C / 2. As many laws are not judgment-based (e.g. tax law), CLAW or LaC should be undertaken to make government services more lawful.
Additionally, people and artificial intelligence (“AI”) should work together and play to our respective strengths to create an augmented, rather than automated, workforce.
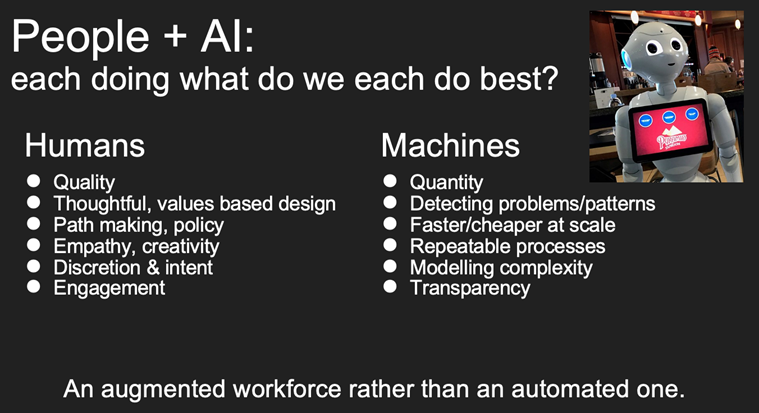
Furthermore, in the specific context of governance, Andrews’s research revealed that the following five AI usage patterns aid to support more nuanced governance – analysis or patterns, automation, content or software generation, automated decision making (e.g. Alexa), and augmentation of work or life (e.g. Siri). Following this, Andrews assessed each of these five AI usage patterns in terms of their suitability of adopting a rules-based or machine learning-based (“ML”) approach. Although Andrews advocates for the ML approach, she notes that this approach may encounter problems relating to traceability and explainability as attributable to Administrative Law and the Rule of Law. For instance, who will be responsible and accountable for the changes in a machine’s decisions as it learns over time? As the concept of “garbage-in-garbage-out” applies in data-based analysis for decision making, the ML approach may be prioritising money and efficiency over people because it lacks a human-based and principles-based approach.
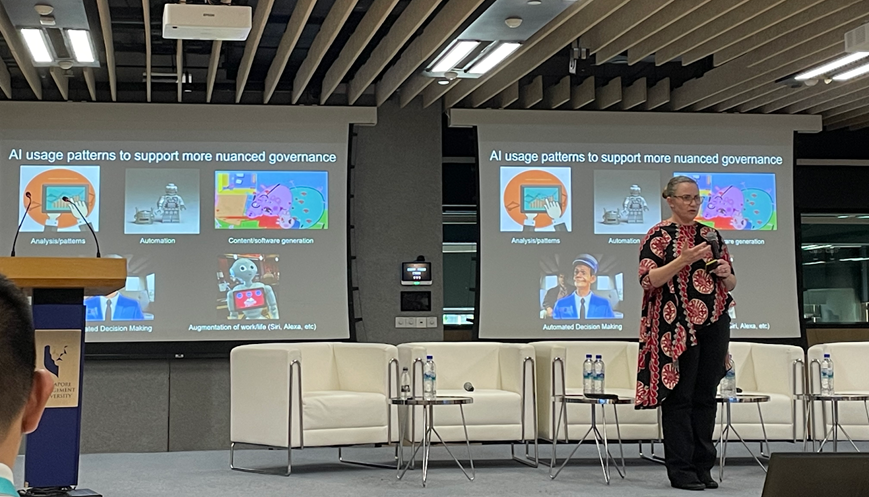
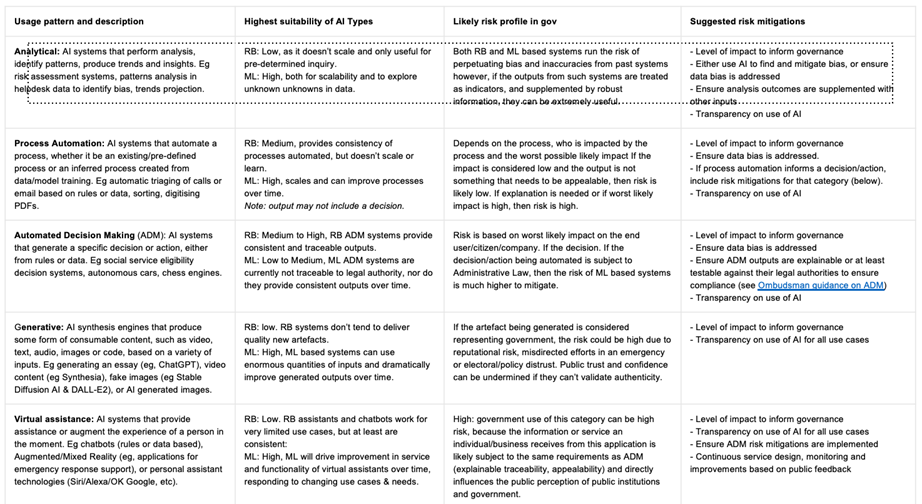
Andrews proposes the following tips to make government services lawful:
- Systems can consume legislation/regulation as code.
- Publicly available machine readable test suite.
- Publicly available modelling tools.
- Decisions are recorded with reasons, available to users.
- Outputs and outcomes are monitored for bias.
- Independent oversight to assure appropriate incentives.
- Rules based, explainable, traceable & testable automated decision making.
(4) How a shared policy infrastructure might help policy management become more adaptive
Fourth, the policy lifecycle must be adaptive to achieve continuous improvement of policy delivery through feedback loops. The creation of a “shared policy infrastructure” is also instrumental in ensuring that policy management remains adaptive.
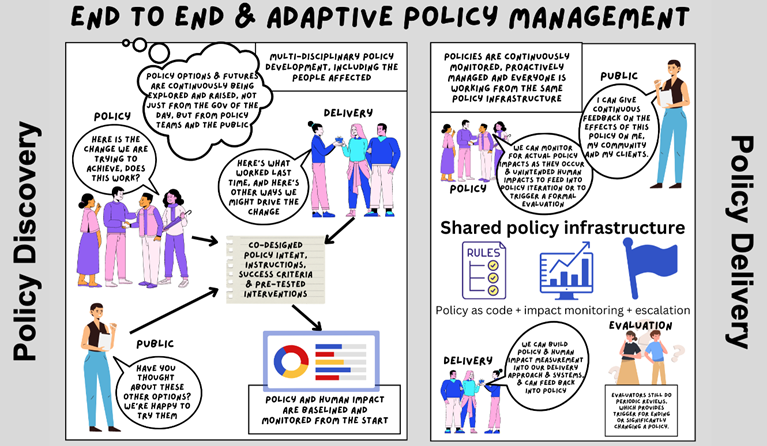
Andrews proposes the following tips to make policy management adaptive:
- Whole of policy lifecycle management.
- Shared policy infrastructure (including the idea of a “policy twin”).
- All policy interventions overseen by policy program.
- Feedback loops to drive continuous improvement.
- Proactive continuous exploration of policy futures.
- Close the gap between policy design and delivery.
(5) How a shared policy infrastructure might help policy delivery be more humane?
Fifth, it is vital to make policy delivery and government services more humane. For example, the Robodebt scheme in Australia “exposed significant cultural, structural, and political issues in how public institutions have administered and managed public policy”,[1] and led to the development of list of recommendations which included, inter alia, the following:[2]
- Recommendation 10.1: Design policies and processes with emphasis on the people they are meant to serve.
- Recommendation 13.2: Feedback processes (from staff).
- Recommendation 17.2: Establishment of a body to monitor and audit automated decision-making.
Furthermore, as per Andrews, “[f]airness of government systems requires measuring for policy AND human impact and active monitoring for & mitigation of harm”. As the intended policy impact may differ from the real-time human impact, there is a need to shift to real-time management of policy impact to unveil any unintended consequences through five steps – measure, monitor, feedback, escalation and iteration.
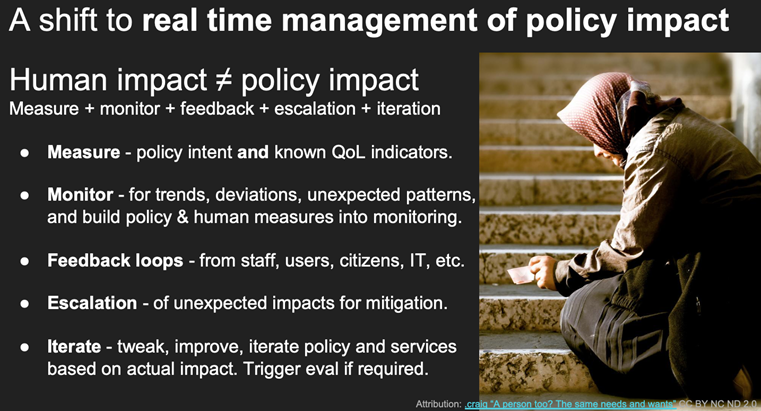
While it is often easy to measure customer service in the private sector because its key performance indicators are heavily based on efficiency, it is difficult to measure customer-citizen experience (“CCX”) because of its added layers of human complexity. For instance, it is difficult to define, let alone measure, terms such as “equitable”, “dignified”, “creepy”, etc as such markers may differ from one person to another. Overall, it is vital to create a “shared policy infrastructure” to produce a good CCX and make policy delivery more humane.
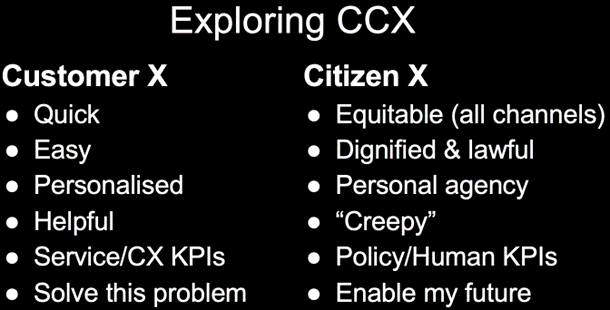
Andrews proposes the following tips to make policy delivery humane:
- Establish shared human measures of success.
- Monitor for & mitigate unintended harms.
- Verifiable claims/credentials as preference to sharing data.
- Funding requests should support human impact.
- Adopt a CCX and omni-channel approach.
- Establish a range of feedback mechanisms (including staff).
- Ensure IT/data/delivery teams are purpose-driven.
- Close interpretation gap with shared policy infrastructure.
Author: Michelle Yap, Alexis N. Chun
Acknowledgement:
This research / project is supported by the National Research Foundation, Singapore under its Industry Alignment Fund – Pre-positioning (IAF-PP) Funding Initiative. Any opinions, findings and conclusions or recommendations expressed in this material are those of the author(s) and do not reflect the views of National Research Foundation, Singapore.
The Technolawgist collaborates with SMU CCLAW in the dissemination of these reflections.